12 Checks for Trustworthy Data Driven Insights
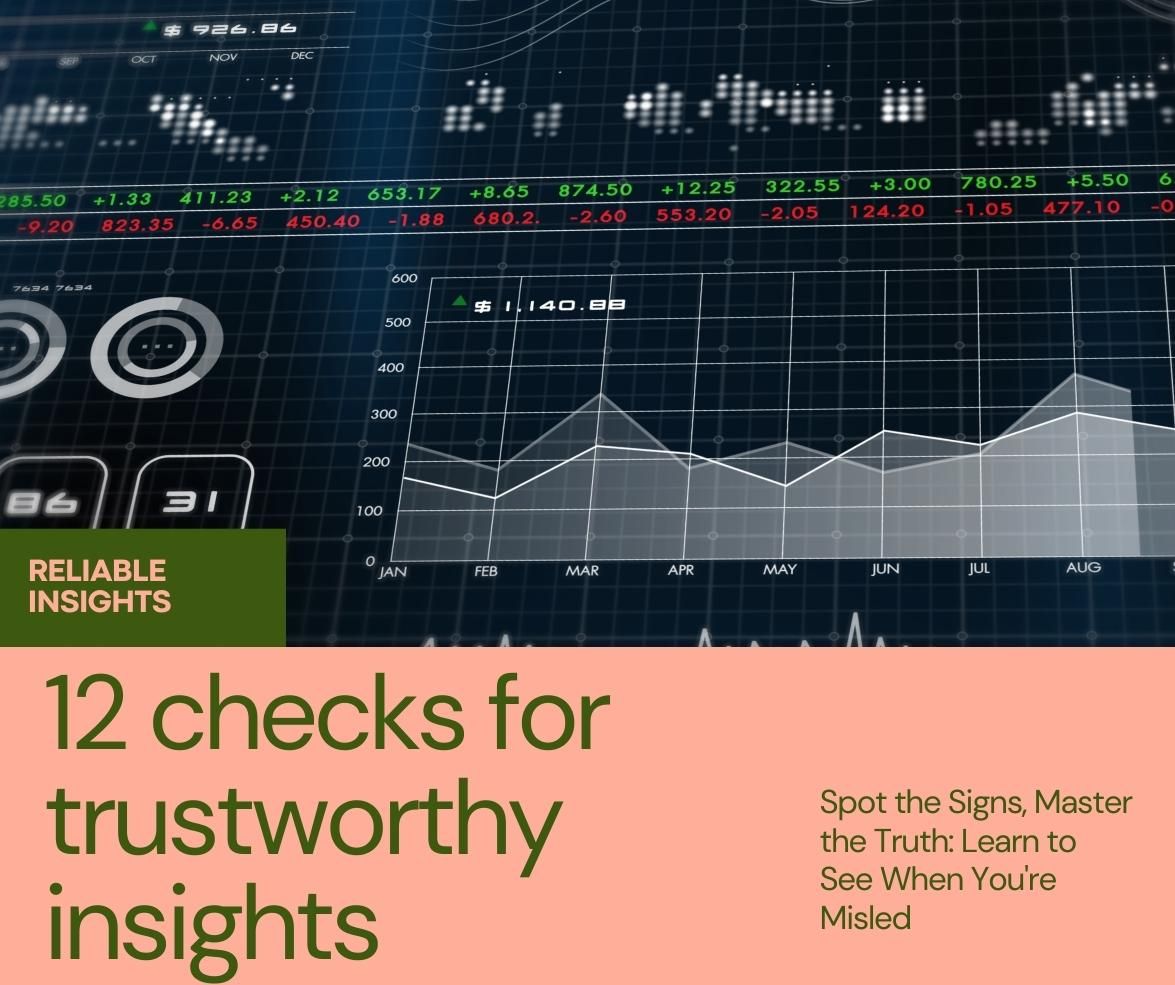
12 Ways to evaluate the integrity of your data insights - Are You Being Misled?
In the quest for data-driven decision-making, insights offer a potent fuel for action, but they can also be a mirage that distorts reality. Not all data whispers the truth—some murmur convenient falsehoods. It's crucial to approach data with a blend of respect and skepticism, recognizing that even the most data-backed conclusions can mislead when the underpinnings are unsound. As you traverse the terrain of information, arm yourself with these 10 incisive tests to challenge the veracity of your data insights. Should you find yourself answering 'Yes' to any of these queries, let that be the signal to dig deeper—because the truth, while sometimes buried, is always worth unearthing.
1. Are there potential conflicts of interest?
When we navigate the landscape of data-driven insights, the compass often points to the biases of those interpreting the data. This isn't just about the data itself; it’s about who is presenting it and why. Analysts, consultants, and even the algorithms that crunch numbers come with their own sets of influences—financial incentives, ideological beliefs, or corporate pressures—that can color the conclusions they draw.
Consider the classic example of tobacco advertising from decades past. A notorious campaign boasted, “More doctors smoke CAMELS than any other cigarette.” This slogan wasn't just a claim; it was a calculated message crafted in an era when the health implications of smoking were downplayed or ignored. It reflects how the interests of tobacco companies—namely, to sell more cigarettes—potentially influenced the message, despite the implications for public health.
This historical case serves as a vivid reminder of the question we must ask of any insight: Are there underlying motivations that could skew the data? Insights should be dissected not just for their content, but also for their context—who is delivering them, what might they gain, and how might this gain tilt the scale of the insight away from the unbiased truth?
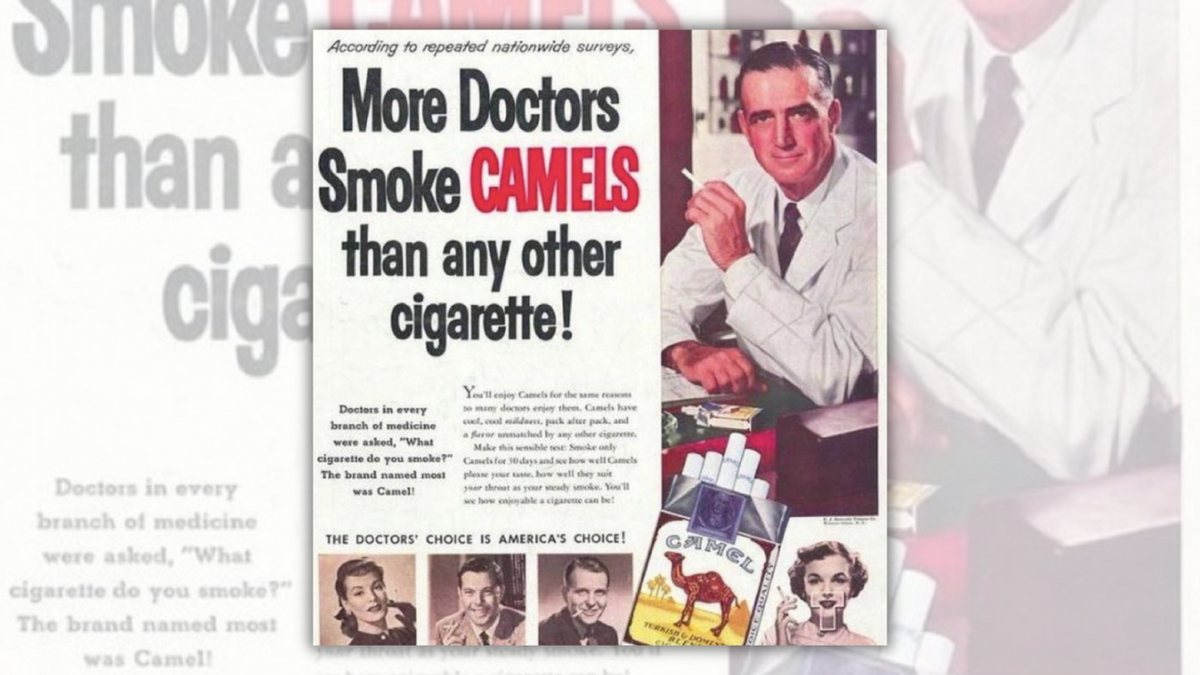
2. Is the data source unclear or questionable?
The journey of data from its origin to the point it becomes an insight is laden with potential pitfalls. The first step in evaluating the robustness of data-driven insights is to trace the data back to its source. Is it derived from a primary, transactional system where data is recorded at the point of action, or has it been subjected to transformations that may obscure its initial state? Consider a common business scenario: data is often pulled into an OLAP cube for reporting purposes. While this facilitates analysis, each transformation step is an opportunity for distortion.
For example, when data from a CRM system is aggregated for marketing insights, discrepancies may arise. The original, unaltered data from the CRM might show detailed customer interactions, but once it’s aggregated, nuances can be lost, and the resultant insights might no longer accurately reflect individual customer behavior. When examining such transformed data, cross-referencing with the raw, untouched figures can often illuminate discrepancies that would otherwise compromise the insight’s credibility.
Moreover, the venue of the data's storage and curation can signal its reliability. Data housed in structured, well-documented databases or repositories that maintain stringent data governance protocols, like a version-controlled database, can generally be considered more reliable than data from opaque systems such as a loosely maintained SharePoint site. The latter can be a quagmire of undocumented changes and uncertain lineage, making it a shaky foundation for high-stakes decision-making.
Therefore, it’s vital to interrogate not just the data but also the context of its sourcing. Is the data fresh from the field, raw and unblemished by interpretation (primary data), or has it been distilled through secondary channels, accumulating layers of potential bias and error? The clarity and directness of your data's provenance are directly proportional to the trust you can place in the insights it generates.
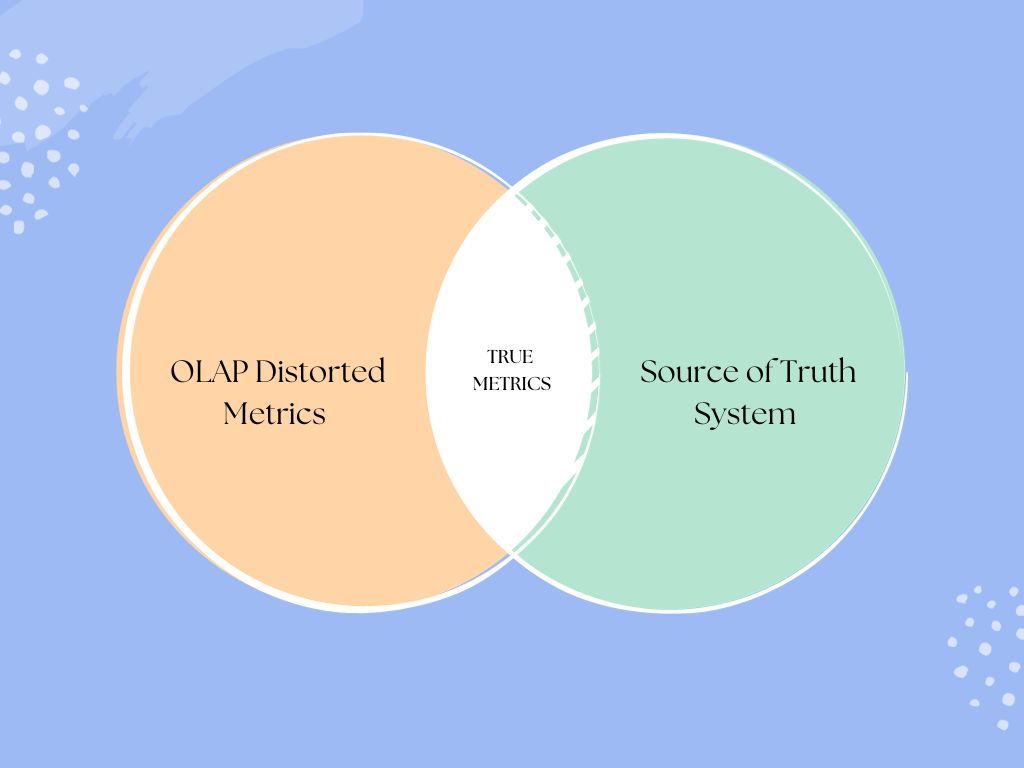
3. Is the message playing to your emotions?
Deciphering whether a message is capitalizing on emotional persuasion—particularly by invoking fear or exploiting the 'Fear of Missing Out' (FOMO)—is essential in the evaluation of data-driven insights. Emotional appeals can be persuasive and motivating, but when they overshadow the factual data, they can lead to skewed decision-making.
One of the most recognized examples in the corporate space that utilized emotional appeal is the 'Y2K' (Year 2000) bug scare. As the new millennium approached, there was widespread panic that computer systems would fail when their clocks rolled over from 1999 to 2000. This fear was rooted in the potential reality of a widespread system breakdown due to a programming shortcut. However, the fervor and urgency to 'fix' the problem were significantly amplified by a fear of the unknown and the possibility of being unprepared for a digital apocalypse. Companies and governments worldwide spent vast amounts of money to update systems, often spurred by the fear of operational collapse rather than a balanced analysis of the actual risk posed by the Y2K bug.
Additionally, FOMO is not just a personal sensation but a strategic corporate lever. In the early days of cloud computing, companies were urged to adopt the technology to avoid being outflanked by more agile competitors. The argument was less about the tangible benefits seen in the data and more about the fear of being left behind in a rapidly changing digital landscape.
When examining insights and their presentations, it is critical to discern whether the core message is fact-based or if it's being embellished by emotional elements to sway opinion. Understanding the data should be the primary driver for decisions, not the emotional weight designed to create urgency or anxiety. This recognition helps in separating emotive noise from insightful signal, ensuring that decisions are made on a foundation of solid evidence.
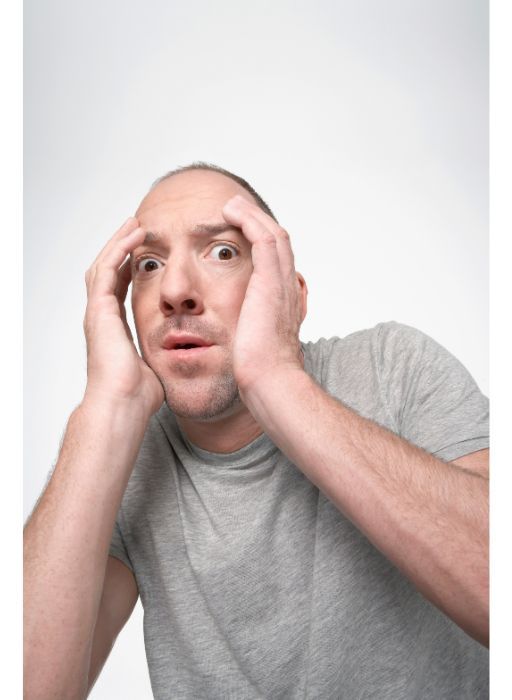
4. Does it rely on small or hidden samples?
The reliability of data-driven insights can be significantly undermined when they are based on limited or non-disclosed sample sizes, as this raises questions about their generalizability and accuracy. This is a well-documented issue in the corporate world, where conclusions drawn from inadequate data samples can lead to misguided strategies and policies.
A notable real-world example of this issue was the launch of New Coke in 1985 by The Coca-Cola Company. The decision was based on taste tests that suggested a sweeter formula was preferred over the original. However, these tests were not only limited in scope, but they also failed to consider the emotional attachment consumers had to the original Coke flavor. The sample used for the market research was not fully representative of Coca-Cola's diverse consumer base, which led to a severe backlash and the eventual return of the original formula as "Coca-Cola Classic."
The New Coke debacle serves as a classic corporate example of the consequences of relying on limited data. It underscores the necessity of thoroughly understanding and disclosing sample size and composition to avoid making far-reaching decisions on potentially unrepresentative data.
Another example, is the influential Minnesota Starvation Experiment involved only 36 men from similar backgrounds. Yet it generated questionable sweeping conclusions about human starvation given the tiny, homogeneous sample.
When insights are based on small or opaque samples, they must be approached with a critical eye, scrutinizing the data for possible biases and limitations that could compromise the integrity of the conclusions.
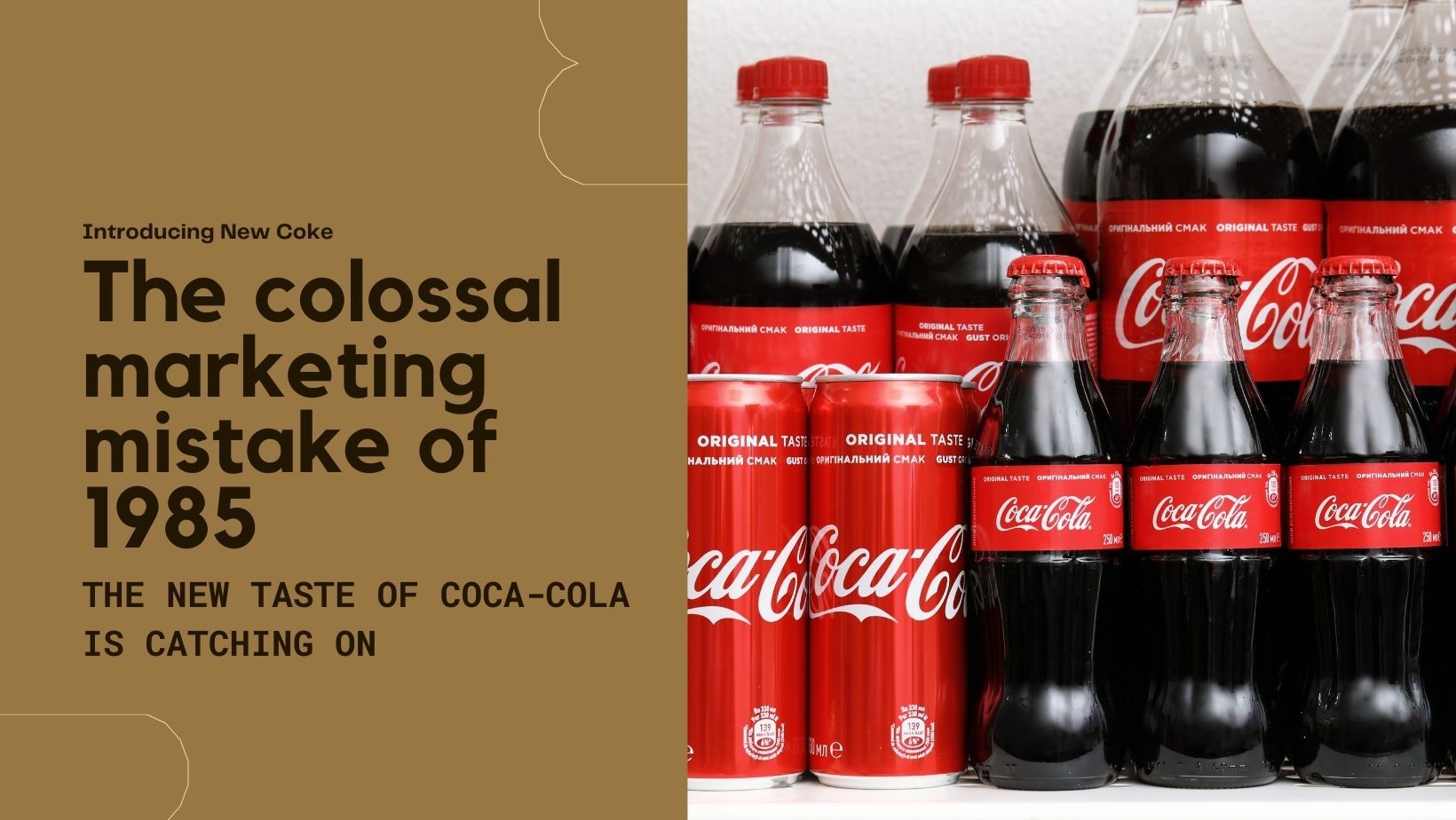
5. Are key terms conveniently redefined?
The redefinition of key terms, particularly within the corporate sector, can significantly influence the perception of performance and management success. This practice is frequently observed in the context of Key Performance Indicators (KPIs), which are often tied directly to bonuses and other forms of managerial incentive.
Let's take the example of customer satisfaction KPIs. Initially, "satisfied customer" may be defined by rigorous standards such as repeat business and direct, positive feedback. However, faced with the potential failure to meet targets, a company might subtly adjust this definition to categorize any customer not actively lodging complaints as "satisfied." This alteration can artificially inflate satisfaction metrics, creating an illusion of performance that doesn't truly exist.
This strategic redefinition serves to present the company in a more favorable light, which can lead to unjustifiable bonuses for executives and a skewed narrative for stakeholders. Such practices undermine the original intent of KPIs as a measure of genuine performance, potentially deceiving investors and the market.
In another vein, the term "vaccine" has undergone a similar transformation. Originally associated with complete immunity, the 2021 update reframed it to emphasize the stimulation of an immune response—a definition more consistent with the effects of COVID-19 vaccines.
Stakeholders must exercise vigilance regarding term redefinitions, especially when these changes align with the timing of performance reviews or bonus evaluations. Upholding a consistent and transparent definition of key terms is vital to preserving the integrity of data insights and to ensuring that incentive structures effectively align with the company’s long-term health and success.
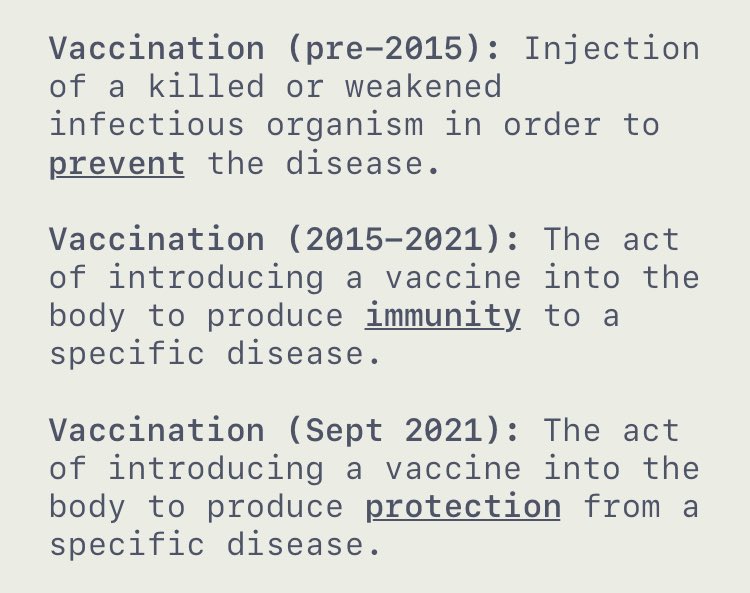
6. Does it silence alternative views?
In the corporate landscape, a prevailing narrative can inadvertently or deliberately suppress competing viewpoints, creating an environment where alternative insights struggle to be heard. The acceptance of data and insights often aligns with prevailing corporate strategies or narratives, while potentially valuable but contradictory information is sidelined or outright ignored.
Consider the pharmaceutical industry's response during the COVID-19 pandemic. Mainstream attention focused on vaccine development, which led to an overshadowing of less prominent, yet potentially significant research, like the correlation between vitamin D deficiency and the severity of COVID-19 symptoms. Despite vitamin D's known role in gene regulation and immune function, its potential as an early intervention strategy did not gain comparable consideration to pharmaceutical options in many professional circles.
This tendency extends to various corporate situations where, for example, innovative but unconventional strategies might be dismissed in favor of tried-and-tested methods, even when new approaches could prove more effective. Such a dynamic can also be observed in financial forecasting, where consensus views often overshadow alternative economic indicators that might provide early warnings or different perspectives on market health.
When insights appear to be excessively aligned with a dominant corporate narrative—especially one that is linked to financial incentives, reputational concerns, or strategic goals—it's crucial to adopt a critical stance. Investigating the sidelined data, questioning the absence of dissenting voices, and examining the motives for prioritizing certain narratives over others are essential steps in ensuring a holistic and balanced understanding of the situation. In doing so, organizations can avoid echo chambers and make well-rounded decisions that consider a full spectrum of perspectives.
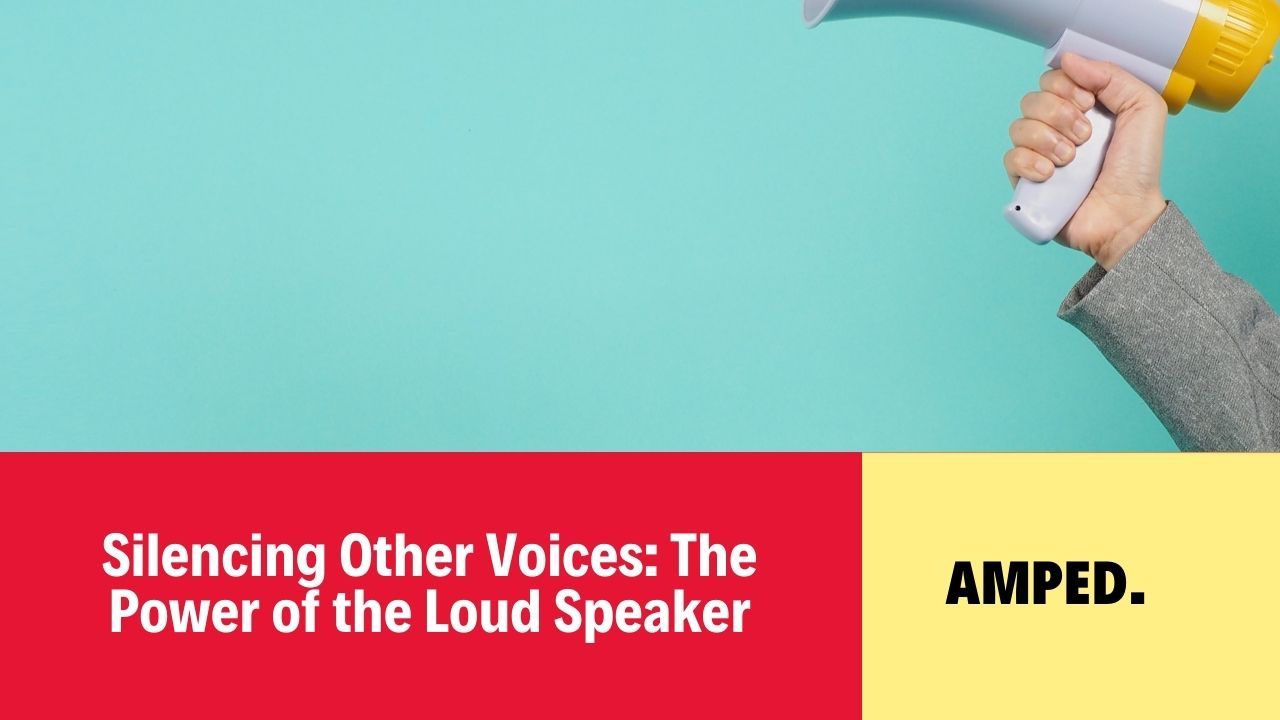
7. Are the metrics cherry-picked or opaque?
The presentation of metrics can often be a ground for factual distortion when the data is selectively curated or details are obscured. This selective presentation can lead to misjudgments and, at times, be a deliberate attempt to deceive.
Consider the pharmaceutical industry, where relative risk reduction is often emphasized over absolute risk reduction to paint a more favorable picture of a drug's effectiveness. For example, a medication might be reported to cut the risk of a certain health event by 50%, a relative figure. In absolute terms, however, this might mean the risk is reduced from 2% to 1%, a mere 1% difference. To an undiscerning eye, the relative reduction number could be misleading, potentially influencing patient and provider decisions.
In a corporate context, this manipulation is similarly observed. A company may report that a new initiative has doubled production efficiency without disclosing that the initial base rate was exceptionally low, thus the actual improvement in absolute terms is minimal. Alternatively, a firm may showcase customer satisfaction rates without clarity on how these are measured, or change the formula for calculating such metrics just before a reporting period to ensure more favorable outcomes, often tied to executive bonuses or stock performance.
Identifying such distortions requires a critical eye to question the context and veracity of the presented metrics. It's essential to demand transparency and complete disclosure to uncover the true story behind the numbers. The discerning stakeholders must recognize when metrics are being manipulated to cast a particular narrative and insist on accessing raw, unaltered data for an accurate assessment.
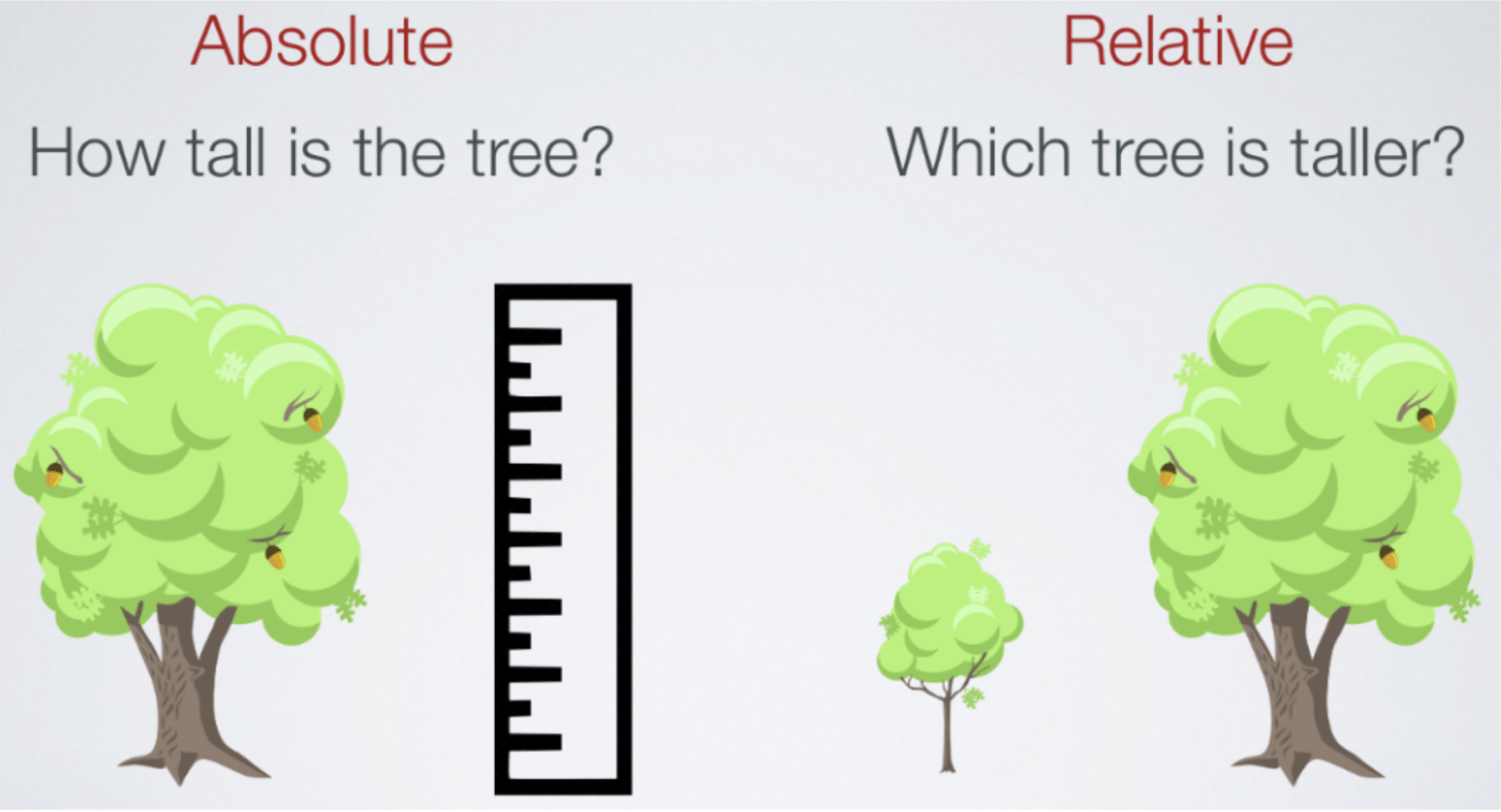
8. Does it confirm pre-existing biases?
When evaluating insights, it's crucial to discern whether they are mirroring biases or genuinely reflecting facts. Confirmation bias can lead to the selective gathering and interpretation of data, molding a narrative that fits pre-established beliefs while neglecting contradicting evidence.
Consider the business sector, where corporate decision-makers might present financial success stories by showcasing certain statistics that affirm their strategy, glossing over figures that indicate underlying problems. This creates a distorted picture of success based on selective truths.
A historical example of this in a more critical context was the presentation of intelligence in the lead-up to the Iraq War. The narrative that Iraq possessed weapons of mass destruction was propagated, with intelligence selectively interpreted to support this view. Evidence that contradicted this narrative, such as the IAEA's findings, was dismissed. This selective presentation of information led to a widely accepted but ultimately false belief that shaped international policy.
Such scenarios underline the importance of examining not just the data presented but also the data omitted. When insights disproportionately affirm a particular view, especially when tied to key decision-makers' beliefs or policies, it's a signal to probe further. Looking beyond the surface to what is not said or shown is essential in identifying when a fact is being misrepresented or when a narrative is being crafted on a foundation of biases rather than balanced evidence.
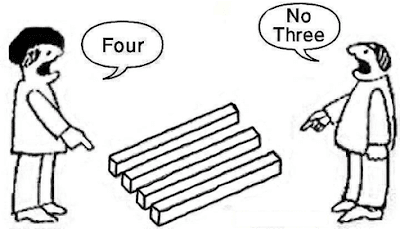
9. The results can't be Independently verified or reproducible?
The robustness of insights hinges on their reproducibility. If independent parties can replicate the process and achieve the same results, the insights are more likely to be reliable. However, this is not always the case, as some findings are presented without the rigors of reproducibility, leading to widespread but unverified conclusions.
Take, for instance, corporate research that claims a new management strategy dramatically boosts productivity. If such studies are proprietary and the methodologies are not shared transparently, they cannot be reproduced or scrutinized by others. This lack of reproducibility means that the insights could be flawed or exaggerated and should be approached with skepticism.
In academia, a notable case involved the link between a gene mutation and chronic fatigue syndrome, where subsequent studies could not replicate the results, pointing to the original's reliance on contaminated samples. This case exemplifies a broader issue in scientific research, often referred to as the "replication crisis," where studies' findings cannot be reproduced by independent research, casting doubt on their validity.
In the corporate context, reproducibility takes on added significance. Stakeholders need to know if claimed results are verifiable and not just one-off claims designed to impress or mislead. If a company's reported insights cannot be tested or repeated by others, it's a red flag. Insights must stand up to independent verification to be considered robust; otherwise, they may merely be anomalies or misconceptions masquerading as credible conclusions.
10. Evidence of coercion in data collection?
Data's reliability can be severely compromised if it is collected under any form of coercion, as this may lead to biased or untruthful responses. Voluntary and genuine participation is critical for the integrity of insights derived from any dataset.
Historically, U.S. census data during the period of slavery is a stark example of such coercion. The enslaved had no voice in this data collection — it was the slaveholders who reported, potentially with motivations to distort information for economic or social advantage. Similarly, authoritarian governments might force citizens to provide favorable responses to surveys under the threat of repercussions, or a company might subtly coerce employees to participate in workplace surveys with the implicit understanding that their careers could be impacted by their responses.
These scenarios lead to a form of response bias where the information gathered does not truly reflect the respondents' experiences or opinions. Instead, it mirrors the outcomes desired by the coercive entity. Such a fundamental flaw cannot be corrected by sophisticated analysis; corrupted data at the source taints all subsequent insights.
Therefore, when evaluating insights, it's imperative to consider the data collection context. If there's any indication that the data may have been gathered under coercion, this must be openly acknowledged, and the data treated with due skepticism. Without confirming the voluntary nature of participation, the insights risk being not just inaccurate, but potentially misleading.
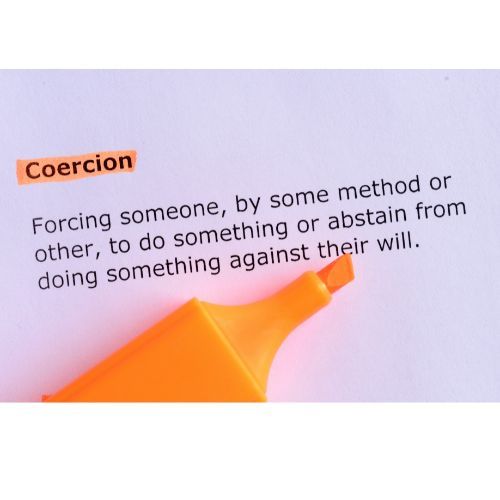
11. Does It overemphasize anecdotal evidence?
Anecdotes can be powerful communication tools, but when they overshadow aggregate data, they may lead to skewed perceptions and insights. Anecdotal evidence often resonates emotionally and can unduly influence how situations are perceived, sometimes more than statistical evidence.
A notable case of anecdotal sway is seen in the daycare abuse panic of the '80s and '90s. A few highly publicized but atypical horror stories of child abuse led to widespread hysteria, causing a disproportionately large number of legal actions against daycare workers. The emotionally charged narratives created a belief in a widespread problem that, statistically, wasn't supported by the overall low incidence rates of verified abuse.
Such dramatic anecdotes, while certainly important in their own right, can distort public perception and decision-making if given undue emphasis over comprehensive data. It's crucial to examine whether these stories are representative or outliers. Robust insights should be grounded in a balanced view that considers both individual narratives and the statistical big picture.
In analyzing insights, it's essential to question whether dramatic stories are being used to overshadow larger, less compelling, but more statistically significant data. A disciplined approach to insight generation will weigh the emotional pull of anecdotes against the empirical weight of aggregated data. This balance ensures a more accurate and nuanced understanding, avoiding the pitfalls of sensationalism.
12. Does It distort through selective timescales?
In the corporate context, the selective presentation of timescales can distort the understanding of business performance or market trends. For instance, a company may present financial results emphasizing a recent surge in profits without acknowledging that this increase is measured against a historically low baseline due to preceding years of decline. This creates an illusion of growth that may not reflect the company's overall health or trend.
Such selective reporting can also occur with productivity metrics or customer growth figures, where the chosen timescale may highlight a temporary positive trend while conveniently excluding periods of stagnation or decline. When reviewing insights, it's important to ask: Are the presented timescales representative of the larger trend, or are they cherry-picked to support a particular narrative?
In decision-making, leaders must ensure that the timescale for any data set provides a comprehensive view that encompasses relevant business cycles and market conditions. Timescale manipulation can mislead stakeholders about the trajectory of the company, possibly resulting in ill-informed strategies and misplaced investments.
Before accepting insights based on timescale-dependent data, verify that the timeframe used is sufficient to understand the full context, including any cyclical behaviors or long-term patterns. Only insights grounded in a fair representation of time will provide the credible foundation necessary for strategic planning and accurate assessment of corporate performance.
Here I have navigated through the labyrinth of potential pitfalls in data interpretation, it's crucial to remember that if you've responded 'yes' to one or more of the checkpoints discussed, it's a signal to pause and probe further. The presence of fallacies in insights isn't just a theoretical concern—it's a practical obstacle to clear and informed decision-making. It's not about being skeptical to the point of inaction but about fostering a culture of thoughtful skepticism that champions the integrity of data. If your data journey hits any of the red flags raised, consider it an opportunity to dig deeper, ask the hard questions, and seek out the full narrative. By doing so, you empower yourself with insights that stand on a foundation of truth, ready to withstand the scrutiny that robust decision-making requires. Join the conversation, share your experiences, and let's collectively sharpen our ability to separate the wheat from the chaff in the world of data insights.
Comments ()